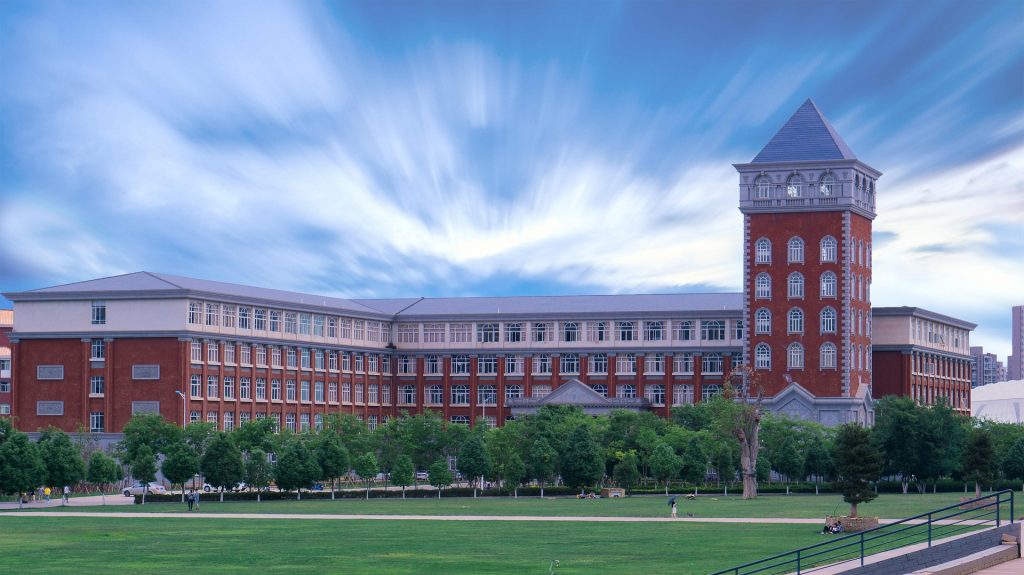
The Effect of University Patent Transfers on Subsequent Innovation: Evidence from Chinese Universities
Abstract
Introduction
Innovation is the cornerstone of a nation’s competitiveness and a fundamental driver of economic growth. Universities, as central hubs of scientific research, play a crucial role in fostering innovation by providing essential research resources. Over the past few decades, China’s investment in university research has increased significantly, rising from 580 million yuan in 1986 to 179.7 billion yuan in 2019. This surge in research funding has led to an exponential increase in research output, particularly in patents.
As research investment grows, universities are producing more scientific and technological outcomes, including academic papers, monographs, and patents. Patents, in particular, have seen a dramatic rise in application numbers. In 2005, Chinese universities filed approximately 20,000 patents annually, a figure that surged to 340,000 by 2019. Notably, around 60% of these patents were invention patents, which typically hold higher technological value. The growing patent output reflects the strengthening role of universities in driving technological advancements and their increasing contribution to innovation.
However, a major challenge lies in the effective transfer and commercialization of university research outcomes. Despite the large number of patents, the actual technology transfer rate remains relatively low compared to developed nations. Recognizing this issue, the Chinese government has implemented several policies, such as the „Action Plan for Promoting the Commercialization of Scientific and Technological Achievements“ issued by the Ministry of Education and other departments between 2016 and 2020. These initiatives aim to facilitate the industrial application of research findings, enhance the efficiency of technology transfer, and accelerate the transformation of scientific discoveries into productive forces.
Given the critical role of universities in national innovation systems, optimizing the process of research commercialization has become an urgent priority. Understanding how university-generated patents transition from research laboratories to real-world applications is essential for fostering economic development and maximizing the societal impact of innovation. Therefore, this study systematically examines the mechanisms and influencing factors behind university patent transfers, providing insights into how policy interventions can enhance technology commercialization and bridge the gap between academic research and industry adoption.
Literature Review
Literature related to university technology transfers
Extensive research has explored the factors influencing university technology transfer. One of the primary determinants is the internal characteristics of universities. The research capacity of an institution, including the size and quality of its research personnel together with the level of R&D investment (Powers 2005, Xu et al. 2011), plays a significant role in facilitating technology transfer. Additionally, the structure and efficiency of university technology transfer offices (TTOs) significantly impact the commercialization process (Siegel et al. 2003a, 2004, Markman et al. 2004). Factors such as incentive mechanisms, managerial efficiency, institutional longevity, organizational scale, and even available resources have an impact on the effectiveness of technology transfer (Markman et al., 2005; Weckowska, 2015). Some studies suggest that well-designed reward systems within universities can positively contribute to technology transfer by motivating researchers to engage (Siegel et al. 2003b, Siegel et al 2004). However, other research indicates that excessive emphasis on financial incentives may divert researchers’ attention from academic entrepreneurship, thereby causing distortion and limiting the broader impact of technology transfer (Grandi and Grimaldi 2005).
The intrinsic attributes of the technology being transferred also determine the likelihood of successful commercialization. Several studies have examined the role of patent characteristics, including the technological significance of innovation, the breadth of patent claims, and the specific domain of expertise (Powers 2005, Bozeman and Gaughan 2007). Moreover, the academic reputation and prior experience of the inventors play a key role in enhancing the credibility and attractiveness of the patented technology to potential industry partners (Chapple et al. 2005).
Another crucial dimension is the interaction between universities and external firms. Effective technology transfer requires strong university-industry linkages. Studies have highlighted the importance of sustained engagement with corporate entities, external licensing agencies, and intermediaries in facilitating the commercialization of research (Siegel et al. 2003a, 2004, Buenstorf and Geissler 2012). The frequency and depth of interactions between academic institutions and industry partners directly influence the speed and success of technology transfer. Additionally, cultural and institutional differences, geographic and economic distance, and discrepancies in technological capabilities or policy environments can create barriers to effective knowledge diffusion (Buenstorf and Schacht 2013, Fang et al. 2014).
While extensive research has examined the determinants of technology transfer, its consequences for subsequent university innovation remain an underexplored area. Theoretically, Thursby and Thursby (2007) applied a life-cycle model, suggesting that under a tenure-track system, revenue from technology transfer should positively influence future research output by providing both financial resources and incentives for continued innovation (Lach and Schankerman 2008). However, empirical evidence presents a more nuanced reality. Studies based on U.S. university data indicate that while financial income from technology transfer tends to reduce the quantity of research output, it positively impacts research quality (Lach & Schankerman). This apparent contradiction has been attributed to the research congestion effect, wherein researchers shift their focus towards deepening and refining existing knowledge rather than producing a high volume of discoveries (Jesen and Thursby, 2003).
Further investigations into academic researchers’ time allocation suggest that engagement in technology transfer reshapes their research agenda. Given their dual responsibilities of conducting research and fulfilling teaching commitments, scholars must navigate trade-offs between fundamental and applied research. Some studies (Jensen and Thursby, 2003) argue that research commercialization incentivizes faculty members to prioritize application-driven projects, which, in turn, may reduce their involvement in exploratory scientific endeavors. These findings highlight the complex interplay between technology transfer, research output, and academic priorities, warranting further empirical investigation into how universities can balance commercialization efforts with fundamental knowledge creation.
Literature related to Patent Transactions
The factors influencing innovation have been extensively studied in the literature. For instance, the stock market is generally more conducive to innovation than the bond market (Hsu et al. 2014), yet its positive impact is constrained by market liquidity (Fang et al. 2014). Similarly, a stable policy environment is more beneficial for fostering innovation than policy interventions themselves (Bhattacharya et al. 2017). Additionally, the establishment of high-tech industrial zones has been found to stimulate innovation through tax incentives, land subsidies, and improved access to financing (Tian and Xu 2022).
However, the ultimate goal of innovation is to realize economic benefits through technological advancements. The circulation of technology plays a crucial role in unlocking its economic potential. Recent research has increasingly focused on technology markets, particularly patent transactions, as a mechanism for reallocating technological resources efficiently. Early studies by Serrano (2010) analyzed patents granted and transferred in the United States, examining transactions from the perspectives of patent ownership, industry affiliation, and patent characteristics. Findings indicate that individual inventors and small firms exhibit the highest patent transaction rates while pharmaceutical and medical-related patents demonstrate the greatest liquidity. Furthermore, factors such as patent age, citation frequency, general applicability, and prior transaction history significantly influence the likelihood of patent transfers.
Building on this foundation, studies by Akcigit et al. (2016) explored the dynamics of U.S. patent markets and revealed that approximately 15–20% of patents are eventually sold, with an average transaction period of 5–6 years. Patents that are technologically distant from their original owner’s core expertise tend to be sold more frequently, with transactions favoring buyers whose technological profile is more aligned with the acquired patents than that of the seller (Ma et al. 2022). Research on firms undergoing financial distress further illustrates the role of patent transactions in corporate restructuring. For instance, Serrano and Ziedonis (2019) examined the sale of patents by companies filing for bankruptcy, finding that firms liquidate a substantial portion of their patent portfolios within the first two quarters following bankruptcy filings, often prioritizing the sale of high-value and strategically significant patents to generate immediate financial relief.
Further empirical research has investigated the role of patent transactions in venture-backed startups. Studies show that among startups in innovation-intensive industries that failed between 1988 and 2008, approximately 70% of their patents were sold, typically within a year of the firm’s closure (Ma et al. 2022). These patents were predominantly acquired by companies operating within the same industry, underscoring the high redistributive value of intellectual property in technology markets. Comparative studies between large and small firms suggest that smaller firms are more active participants in patent transactions. (Figueroa and Serrano 2019). While large firms are more likely to acquire high-value patents due to their capacity for internal technology integration, smaller firms prioritize patents that complement their existing technological portfolios. Transaction costs also play a significant role, as smaller firms exhibit greater flexibility in engaging in patent sales, whereas larger corporations may find internal R&D investments more cost-effective.
Recent studies have begun to explore the broader impact of patent transactions on innovation. Patent transactions serve as a mechanism for optimizing the allocation of technological resources, thereby fostering economic growth and improving social welfare. Empirical evidence suggests that the economic value generated through patent transactions corresponds to approximately 10% of the transferred patent’s intrinsic value and increases as transaction costs decline (Serrano 2018).
At the firm level, research by Brav et al. (2018) found that external financial interventions, such as hedge fund involvement, significantly increase the likelihood of patent sales. Firms tend to divest patents that are technologically distant from their core operations, thereby ensuring that intellectual property is utilized more efficiently and enhancing innovation efficiency. Han et al. (2022) demonstrated that patent transactions not only facilitate corporate innovation but also enable firms to specialize in their core technological competencies, promoting a more structured division of labor in innovation processes.
Additional studies by Hochberg et al. (2018) indicate that patents that are more likely to be transacted also have higher potential as collateral for financial leverage. The ability to liquidate patents enhances their residual value, increasing the feasibility of using patents for secured loans. Furthermore, research on patent litigation suggests that the likelihood of legal disputes decreases following patent transactions, as acquiring firms typically possess stronger legal resources to enforce intellectual property rights and resolve infringement claims more effectively (Galasso et al. 2013).
Research Gaps and Contributions of This Study
Existing research on university technology transfer and patent transactions presents several key limitations. First, most studies focus on the factors influencing university technology transfer, with relatively few examining the transfer of university innovation outcomes from a patent-level perspective (Akcigit et al 2016, Serrano 2018). Second, while prior studies have explored the broader implications of patent transactions, such as their impact on social welfare and firm-level performance, their effects on universities remain underexplored (Han et al 2022, Brav et al. 2018, Galasso 2013). Given that universities are major innovation hubs, understanding how university research activities are influenced by patent transfers is both a significant and pressing issue. This study addresses this gap by investigating university technology transfer through the lens of patent transactions and examining its impact on subsequent university innovation.
This study contributes to the literature on university technology transfer and innovation by addressing several key gaps and extending prior research in the following three aspects:
- Investigating the Impact of Patent Transfers on University Innovation
While prior studies have explored the factors influencing university technology transfer, relatively few have examined how patent transfers—an essential mechanism of technology commercialization—affect subsequent university innovation. By analyzing patent-level data, this study provides empirical insights into whether and how patent transactions stimulate further innovation within universities.
- The Role of Faculty Qualifications in Technology Transfer and Innovation
Existing research suggests that the quality of university researchers plays a crucial role in technology commercialization, but limited attention has been given to its moderating effect on the relationship between patent transfers and innovation. This study hypothesizes that universities with a higher proportion of senior faculty members—who possess greater expertise, credibility, and industry recognition—are more likely to experience stronger positive effects of patent transfers on subsequent innovation.
- The Influence of University-Industry Social Networks
Technology transfer is inherently influenced by the strength of university-industry relationships, as close ties facilitate knowledge exchange and reduce information asymmetry in technology commercialization. This study examines whether universities with richer industry networks benefit more from patent transfers, leading to greater innovation outcomes.
The paper is structured as follows: Section 3 presents the data. Section 4 outlines the empirical model, detailing the methodology employed to examine the relationship between patent transfers and university innovation. Section 5 discusses the results, providing empirical findings and interpretations. Finally, Section 6 concludes the study, summarizing key insights and policy implications.
Data
This study utilizes two primary datasets. The first dataset consists of patent transfer data spanning from 1999 to 2016, which includes core information such as patent application numbers, assignors, assignees, and execution dates of the transfers. The second dataset comprises basic patent information, covering patents that were applied for and granted between 1985 and 2016. This dataset includes details such as application numbers, applicants, grant dates, and application dates. Both datasets were obtained from the China National Intellectual Property Administration (CNIPA). Additionally, patent-level data from the EPS Database and CSMAR Database are incorporated as supplementary sources to enhance cross-validation and ensure robustness in the analysis.
Data Pre-Processing
To ensure the accuracy and reliability of the patent transfer data and to exclude non-commercial transfers, this study applied a series of preprocessing steps. The patent transfer dataset was first merged with the basic patent information dataset using patent application numbers, retaining only patents originally filed by domestic universities. Observations with missing key information, such as assignors, assignees, or execution dates, were removed. Additionally, records where the execution date of the transfer exceeded the patent’s expiration date, or where the patent lifespan was abnormally short (less than four years) or beyond the maximum statutory validity, were excluded to prevent inconsistencies. Duplicate transactions involving the same patent being transferred multiple times by the same assignor or received multiple times by the same assignee were also removed. Given that not all registered patent transfers represent market-driven technology transactions, non-commercial transfers were identified and eliminated based on established methodologies in the literature, with adjustments made to fit the specific characteristics of the dataset. Following these data-cleaning procedures, the final dataset comprises university-originated patent transfers that occurred between 1999 and 2016.
Summary Statistics
This section presents descriptive statistics for the key variables in the regression sample, as shown in Table 1. The dataset consists of 8,705 university-year observations spanning from 1999 to 2016.
On average, universities apply for and are granted approximately 125.87 patents per year, but there is substantial variation across institutions, as indicated by a standard deviation of 212.61. Some universities record no patent applications in certain years, while the most productive institutions apply for as many as 1,165 patents in a single year. Among these granted patents, invention patents account for more than half, with a mean of 67.33, while utility model patents are slightly lower at 55.24. Notably, the distribution of invention patents exhibits greater variability across universities compared to utility models.
Regarding patent liquidity, the average probability of a university patent being transferred is 1.07%, with invention patents (1.25%) being more likely to be transacted than utility model patents (0.79%). This suggests that invention patents, which typically involve more substantial technological advancements, have greater marketability and higher demand in technology transfer.
In terms of university resources, the average annual R&D expenditure per university is approximately $17.36 million, with some institutions investing as much as $241.92 million, while others report little to no spending. Similarly, the number of researchers per university averages 411, but varies significantly, ranging from 3 to 3,893. University size, measured by the total number of students, faculty, and staff, exhibits considerable dispersion, with an average of 1,157.99 and a maximum of 9,376.
Additionally, in the university patent transfer dataset, 83.61% of all transfers occur after the patent has been granted, meaning that the analysis focuses primarily on post-grant patent transfers (patent rights transfers), rather than pre-grant transfers (application rights transfers). Given the nature of patent commercialization, universities appear to engage more actively in patent transactions after securing official patent rights.
Table 1. Summary Statistics | ||||||
Variables | Mean | SD | Min | Median | Max | Sample Size |
Number of patents | 125.87 | 212.61 | 0 | 40 | 1165 | 8705 |
Number of inventions | 67.33 | 139.75 | 0 | 12 | 836 | 8705 |
Number of utilities | 55.24 | 97.68 | 0 | 16 | 569 | 8705 |
Liquidity (%) | 1.07 | 0.54 | 0 | 1.18 | 2.35 | 8705 |
Liquidity_invention (%) | 1.25 | 0.79 | 0 | 1.43 | 3.05 | 8705 |
Liquidity_utility (%) | 0.79 | 0.46 | 0 | 0.90 | 1.76 | 8705 |
R&D expenditure (M) | 17.36 | 38.36 | 0 | 3.36 | 241.92 | 8705 |
Number of researchers | 411.28 | 598.26 | 3 | 215 | 3893 | 8705 |
Scale/University size | 1157.99 | 1490.13 | 46 | 718 | 9376 | 8705 |
Note: Scale/University size is measured by the total number of faculty and staff. R&D expenditure is measured by millions of dollars.
Patent Type
The composition of university patent transfers from 2001 to 2015 reveals a strong dominance of invention patents, which consistently accounted for the majority of transferred patents. In 2001, all transferred patents were invention patents (100%). Although the share fluctuated over time, it remained consistently high, averaging around 85% in most years. The lowest recorded proportion of invention patents occurred in 2004 (54.05%), coinciding with a temporary increase in utility model patents, which accounted for 40.54% of transfers that year.
Utility model patents generally constituted a smaller but stable share, ranging between 10% and 17% in most years, except for notable peaks in 2004 (40.54%) and 2015 (14.29%). Design patents, on the other hand, represented the smallest fraction of transferred patents, exceeding 5% only in 2004 (5.41%), while in most years, their share was negligible or zero.
The consistently high proportion of invention patents in university technology transfer suggests that universities primarily commercialize patents with higher technological and commercial value, aligning with their role as key innovation hubs. This trend also indicates that university patent transfers emphasize knowledge-intensive technologies, reinforcing their significance in driving technological progress and industry applications.
Table 2. Type Composition of Patent Sample | |||
Inventions | Utilities | Designs | |
2001 | 100 | 0 | 0 |
2002 | 95.83 | 0 | 4.17 |
2003 | 92.86 | 7.14 | 0 |
2004 | 54.05 | 40.54 | 5.41 |
2005 | 75.76 | 24.24 | 0 |
2006 | 87.32 | 11.27 | 1.41 |
2007 | 87.62 | 12.38 | 0 |
2008 | 81.70 | 17.67 | 0.63 |
2009 | 82.83 | 17.17 | 0 |
2010 | 86.78 | 13.22 | 0 |
2011 | 83.89 | 16.00 | 0.12 |
2012 | 85.96 | 13.96 | 0.08 |
2013 | 86.61 | 12.89 | 0.50 |
2014 | 89.35 | 9.93 | 0.71 |
2015 | 85.51 | 14.29 | 0.19 |
Industry Composition
The industry distribution of university patent transfers from 2001 to 2015 highlights a strong concentration in the manufacturing sector, which consistently accounted for the largest share of transferred patents. In the early years, manufacturing patents made up nearly 100% of all transfers, with 2001, 2002, and 2005 seeing no transfers in any other sector. Over time, the dominance of manufacturing patents declined slightly, reaching 86.19% in 2015, indicating a gradual diversification in university technology commercialization.
The second-largest category of transferred patents belonged to the information transmission, software, and information technology services sector, which saw a steady increase in its share over time. While its proportion remained below 10% in most years, it reached 8.53% in 2012 and continued to grow, reflecting the increasing role of digital and software-related innovations in university technology transfer.
Other industries, such as electricity, heat, gas, and water production and supply, as well as construction, accounted for relatively small but gradually rising shares. For instance, patents in the electricity and energy sector increased from 0% in the early 2000s to nearly 5% by 2015, signaling growing university engagement in energy-related innovations. Similarly, agriculture, forestry, animal husbandry, and fishery, as well as mining, had only minimal participation in patent transfers, with their combined share rarely exceeding 1-2% in any given year.
These trends suggest that while manufacturing remains the dominant sector for university patent transfers, there is a clear shift towards greater industry diversification, with increasing participation from the technology, energy, and infrastructure sectors. This evolution reflects the broader transformation of university research commercialization, as more industries engage in the adoption and application of academic innovations.
Table 3. Industry Composition of Patent Trading Sample | ||||||
Year | Agriculture, Forestry, Animal Husbandry, and Fishery | Mining | Manufacturing | Electricity, Heat, Gas, and Water | Construction | Software and Information Technology Services |
2001 | 0 | 0 | 100 | 0 | 0 | 0 |
2002 | 0 | 0 | 100 | 0 | 0 | 0 |
2003 | 7.14 | 0 | 92.86 | 0 | 0 | 0 |
2004 | 0 | 0 | 97.33 | 0 | 0 | 2.70 |
2005 | 0 | 0 | 100 | 0 | 0 | 0 |
2006 | 0 | 2.82 | 80.28 | 1.41 | 0 | 15.49 |
2007 | 2.86 | 0 | 92.38 | 0 | 0 | 4.76 |
2008 | 0.63 | 0.32 | 89.27 | 2.84 | 0.63 | 6.31 |
2009 | 0.76 | 0.51 | 90.15 | 1.77 | 2.53 | 4.29 |
2010 | 1.11 | 0 | 90.31 | 2.64 | 2.86 | 3.08 |
2011 | 1.42 | 0.12 | 91.35 | 1.90 | 1.54 | 3.67 |
2012 | 0.93 | 0 | 85.73 | 3.34 | 1.47 | 8.53 |
2013 | 0.60 | 0.15 | 89.89 | 2.49 | 1.44 | 5.43 |
2014 | 1.28 | 0.19 | 89.83 | 1.76 | 1.14 | 5.80 |
2015 | 0.97 | 0.05 | 86.19 | 4.97 | 1.55 | 6.28 |
Model
Variables
The key explanatory variable in this study is patent liquidity, which captures the likelihood of a university’s patents being transferred. Since patent transfers and university innovation may be endogenously related, this study constructs a patent liquidity index to mitigate potential biases. This measure follows existing methodologies but is adapted to fit the dataset’s specific characteristics. Patent liquidity is defined as the average probability of a university’s valid patents being transacted (Serrano 2010). To construct this measure, the transaction probability of each patent is estimated based on its authorization year, industry classification, and the year of transfer. Specifically, for each year, the transaction probability is calculated as the proportion of patents within a given authorization year and industry category that were successfully transferred. Since each patent has a corresponding authorization year and industry classification, this approach allows for the estimation of transaction probabilities across universities.
The stock of valid patents at each university includes only those that have been granted but have not yet expired. Given that most patent transfers occur within a few years after authorization, this study considers only patents authorized within the past six years when constructing the university’s valid patent stock. Empirical distributions indicate that more than 70% of patent transfers take place within the first six years after authorization. Additionally, because design patents typically have lower technological content and account for only a small fraction of transactions in the dataset, this study focuses on invention patents and utility models when examining the impact of patent transfers on innovation. The patent liquidity index is thus computed as the average probability of valid patents being transacted at each university, with separate calculations for invention patents and utility models to allow for heterogeneity analysis.
Using patent liquidity as the explanatory variable offers two key advantages. First, it is directly linked to actual patent transfer activity, as it is constructed using real transaction data while accounting for authorization year and industry-specific characteristics. A higher patent liquidity index implies a greater likelihood of patent transfers at a given university. Second, it reduces concerns regarding potential endogeneity, as the index is derived from the full patent transaction dataset rather than being influenced by any single university’s characteristics. This ensures that the measure remains largely exogenous to individual university-level factors, mitigating biases related to reverse causality.
The dependent variable in this study is university innovation output, measured as the number of patents applied for and granted by each university. Following established literature, granted patent counts serve as a proxy for innovation performance. To account for the skewed distribution of patent filings, the negative binomial model is used. Similar measures are also constructed for invention patents and utility models. Given that innovation processes take time, patent applications are expected to exhibit a lagged response to influencing factors. Therefore, a two-year lag of patent counts is used as the primary dependent variable, with additional robustness checks conducted using a one-year lag to validate the results.
To control factors that may also influence university innovation output, several variables are included in the regression models. These include R&D expenditures, the number of research personnel, and university size, where university size is proxied by the total number of faculty members. Each of these variables is log-transformed to improve model stability. The data for these controls are sourced from the Compilation of Science and Technology Statistics for Higher Education Institutions.
Endogeneity
This study incorporates university-fixed effects and year-fixed effects into the regression model. University fixed effects control for time-invariant institutional characteristics that may influence both patent liquidity and innovation, such as university reputation, historical research strength, or long-standing industry collaborations. Year-fixed effects account for macroeconomic and policy changes that could impact university innovation trends over time, such as national R&D funding policies, intellectual property (IP) law reforms, or broader shifts in technology markets. Together, these fixed effects help eliminate unobserved heterogeneity that could bias the estimation results.
A key concern in assessing the causal impact of patent liquidity on university innovation is reverse causality—that is, universities that are inherently more innovative may engage in more technology transfers rather than technology transfers driving future innovation. To address this issue, this study adopts a lagged explanatory variable approach, using one-year lagged patent liquidity as the key independent variable. This approach aligns with the theoretical expectation that patent commercialization activities today influence research and innovation outcomes with a time lag, rather than the other way around. By ensuring that patent liquidity at time t is used to explain innovation output at time t+1, the model reduces the risk of simultaneity bias.
Additionally, to further eliminate potential endogeneity, this study employs an instrumental variable (IV) approach, using the total technology transfer volume in the province where a university is located (excluding the university’s transactions) as an instrument for patent liquidity. The rationale for this IV is that technology transfer activity at the provincial level reflects the overall vibrancy of the regional technology market, which likely influences the ease and likelihood of a university transferring its patents. However, this measure is unlikely to directly impact a specific university’s patenting decisions beyond its effect on liquidity. The assumption is that while a dynamic regional technology market facilitates knowledge diffusion and enhances the probability of patent transactions, it does not independently drive a university’s innovation output.
By incorporating fixed effects, lagged explanatory variables, and an IV approach, these methodological choices help ensure that the estimated effects are not driven by omitted variable bias, simultaneity, or unobserved regional shocks. Robustness checks, including alternative lag structures and placebo tests, further validate the reliability of the findings.
Empirical model specification
To exame the impact of patent transfers on universitllowing empirial model:
The empirical model in this study examines the relationship between patent transfers and university innovation. I use the negative binomial model since the number of patents is a count variable and can be zero in our data set. In the model, i represents universities, and t denotes years. The key explanatory variable is patent liquidity, which measures the probability of a university’s patent being transferred. The dependent variable is the log-transformed count of patents applied for and granted, with a one-year lag to account for the time required for research and development before patents are filed and approved.
The regression also includes several control variables that may influence university innovation, including R&D expenditures (ln R&D), research personnel (ln Researchers), and university size (scale). To account for unobserved heterogeneity, university-fixed effects, and year-fixed effects are incorporated into the model. Standard errors are clustered at the university level to correct for potential serial correlation. Additionally, to mitigate the influence of extreme values, continuous variables are winsorized at the 1st and 99th percentiles.
Results
Baseline Estimation
This section presents the baseline regression results examining the impact of patent liquidity on university innovation output. Table 4 reports results from three different specifications: instrumental variable (IV) estimation, fixed effects (FE) estimation, and ordinary least squares (OLS) estimation. Across all models, patent liquidity is found to have a positive and statistically significant effect on innovation, reinforcing the hypothesis that a more active patent market enhances university research output.
Column (1) presents results from the IV estimation, addressing potential endogeneity concerns by using the total technology transfer volume in the province (excluding the university’s transactions) as an instrument for patent liquidity. The estimated coefficient for patent liquidity (0.125, p < 0.01) remains positive and statistically significant, suggesting that an increase in university patent market activity stimulates further innovation.
Column (2) reports the FE model, which includes university-fixed effects and year-fixed effects to account for unobserved time-invariant institutional characteristics and broader economic or policy changes that could influence university innovation trends. The coefficient on patent liquidity (0.136, p < 0.01) remains robust, supporting the argument that increased patent transfers are associated with higher research output, even after controlling university-specific factors.
Column (3) presents the OLS regression, which, while yielding a larger coefficient (0.633, p < 0.01), is more susceptible to omitted variable bias and potential simultaneity issues. The discrepancy in coefficient magnitudes across specifications suggests that OLS may overestimate the effect of patent liquidity on innovation due to endogeneity concerns.
Table 4. The Effect of Patent Liquidity on Innovation | |||
(1) | (2) | (3) | |
IV | FE | OLS | |
Liquidity | 0.125*** | 0.136*** | 0.633*** |
(0.05) | (0.05) | (0.07) | |
Ln(R&D) | 0.022*** | 0.027*** | 0.057*** |
(0.00) | (0.006) | (0.01) | |
Ln(researchers) | 0.135*** | 0.142*** | 0.470*** |
(0.04) | (0.050) | (0.06) | |
scale | -0.118*** | -0.097*** | 0.364*** |
(0.04) | (0.048) | (0.10) | |
University FE | Yes | Yes | No |
Year FE | Yes | Yes | No |
1-stage F statistics | 14.72 | – | – |
Adjusted | 0.855 | 0.468 | 0.215 |
Observations | 8705 | 8705 | 8705 |
Note: The dependent variable is the number of patents. The estimation is conducted using a negative binomial model. Adjusted R-squared values are reported to assess model fit. ***p < 0.01, **p < 0.05, *p < 0.1.
Differentiated Effects by Patent Type
Since invention patents dominate university patent transfers and exhibit higher liquidity than utility models (Table 2), it is crucial to examine whether the impact of liquidity varies across patent types. Table 5 presents separate estimations of the effect of invention patent liquidity on invention and utility while controlling for fixed effects and other covariates.
The results indicate that utility model innovation is more responsive to changes in liquidity. Specifically, a one-standard-deviation increase in liquidity leads to a 38.6% increase in utility model output, whereas the corresponding increase for invention patents is 16.5%. This suggests that utility models, which are typically less technologically complex, respond more immediately to fluctuations in patent liquidity.
One possible explanation for this pattern is that utility models tend to have shorter commercialization cycles and lower transaction costs, making them more sensitive to shifts in the patent transfer market. In contrast, invention patents often involve longer R&D processes, higher technological complexity, and more stringent approval requirements, leading to a more gradual response to changes in liquidity. This aligns with the notion that high-tech patents require sustained investment and industry collaboration, whereas utility models are often more readily commercialized by firms seeking incremental innovations.
Table 5. The Effect of Patent Liquidity on Innovation: Different Types of Patents | |||
(1) | (2) | (3) | |
Patents | Inventions | Utilities | |
Liquidity | 0.125*** | 0.165*** | 0.386*** |
(0.05) | (0.03) | (0.06) | |
Ln(R&D) | 0.022*** | 0.018*** | 0.026*** |
(0.00) | (0.00) | (0.01) | |
Ln(researchers) | 0.135*** | 0.067** | 0.121** |
(0.04) | (0.03) | (0.05) | |
scale | -0.118*** | -0.146** | -0.236** |
(0.04) | (0.07) | (0.09) | |
University FE | Yes | Yes | Yes |
Year FE | Yes | Yes | Yes |
IV | Yes | Yes | Yes |
Adjusted | 0.855 | 0.897 | 0.807 |
Note: The dependent variable is the number of patents, inventions and utilities. The estimation is conducted using a negative binomial model. ***p < 0.01, **p < 0.05, *p < 0.1.
Heterogeneous Effects by University Type
The impact of patent liquidity on innovation varies across university types, given their distinct research orientations and industry links. Unlike firms that operate within specific industries, universities are classified based on their disciplinary focus. Following the Shanghai Ranking’s classification, this study categorizes universities into comprehensive, science & engineering, and medical universities and examines how patent liquidity affects innovation within each group (Table 6).
The results show that patent liquidity positively affects innovation across all university types, reinforcing the role of an active patent market in fostering knowledge commercialization. Among them, science & engineering universities exhibit the strongest response to invention patent liquidity (0.238*), suggesting that technology-driven institutions benefit most from dynamic patent markets due to their stronger industry collaborations and applied research focus. For utility models, comprehensive universities show the highest sensitivity (0.395*), indicating that multidisciplinary institutions may be more engaged in commercializing lower-complexity patents. In contrast, medical universities show the weakest response to patent liquidity, particularly for utility models (0.272*), likely due to longer commercialization timelines and regulatory constraints in medical research.
Table 6. The Effect of Patent Liquidity on Innovation: Different Types of University | ||||||
(1) | (2) | (3) | (4) | (5) | (6) | |
Univ. Type | Comprehensive | Science & Engineer | Medical | |||
Patent Type | Inventions | Utilities | Inventions | Utilities | Inventions | Utilities |
Liquidity | 0.160*** | 0.395*** | 0.238*** | 0.394*** | 0.181** | 0.272* |
(0.05) | (0.13) | (0.06) | (0.14) | (0.07) | (0.14) | |
Ln(R&D) | 0.017** | 0.041*** | 0.009 | 0.006 | 0.023 | 0.024 |
(0.01) | (0.01) | (0.01) | (0.01) | (0.02) | (0.02) | |
Ln(researchers) | 0.045 | 0.105 | 0.082 | 0.111 | -0.108 | -0.093 |
(0.06) | (0.11) | (0.05) | (0.09) | (0.13) | (0.15) | |
scale | -0.90 | -0.208 | 0.047 | 0.348 | -0.128 | -0.025 |
(0.13) | (0.18) | (0.20) | (0.29) | (0.14) | (0.18) | |
University FE | Yes | Yes | Yes | Yes | Yes | Yes |
Year FE | Yes | Yes | Yes | Yes | Yes | Yes |
IV | Yes | Yes | Yes | Yes | Yes | Yes |
Adjusted | 0.917 | 0.812 | 0.897 | 0.765 | 0.753 | 0.618 |
Observations | 1503 | 1503 | 1717 | 1717 | 538 | 538 |
Note: The dependent variable is the number of inventions and utilities. The estimation is conducted using a negative binomial model. ***p < 0.01, **p < 0.05, *p < 0.1.
Heterogeneous Effects of Patent Liquidity on University Innovation
As highlighted in the literature, the actual occurrence of patent transfers is influenced by multiple factors, among which university-specific characteristics play a crucial role. Two particularly important aspects are the qualifications of university researchers and their social networks. These factors shape the ability of universities to effectively transfer technology and determine how patent liquidity translates into innovation outcomes.
The Role of Researcher Qualifications
Since technology is an intangible asset, it is often characterized by high information asymmetry and significant uncertainty regarding its market potential and economic value. If a technology is developed by researchers with higher academic qualifications, it is likely to be perceived as more valuable, making it easier to commercialize. To capture this dimension, this study measures the share of senior faculty members (with high academic ranks) within each university over the sample period. Universities with a share above the median are classified as high-research-qualification institutions, while those below the median are considered low-research-qualification institutions.
Table 7 presents the estimation results, showing that higher researcher qualifications strengthen the effect of patent liquidity on innovation across both invention and utility model patents. Specifically, in universities with high research qualifications, a one-standard-deviation increase in patent liquidity leads to a 12.5% increase in invention patent output and a 51.7% increase in utility model output. In contrast, in universities with lower research qualifications, the corresponding effects are only 11.3% and 24.4%, respectively. These findings suggest that universities with more highly qualified researchers benefit more significantly from an active patent market. Highly qualified researchers may have greater technical expertise, stronger reputations, and broader professional recognition, which enhances the perceived value and marketability of their patents. Moreover, universities with more senior faculty members may have more developed internal support mechanisms for patent commercialization, further reinforcing the positive effects of patent liquidity on innovation output.
Table 7. The Heterogeneous Effects of Patent Liquidity on Innovation by Researchers’ Qualifications | ||||
High Research Qualifications | Low Research Qualifications | |||
Inventions | Utilities | Inventions | Utilities | |
Liquidity | 0.205*** | 0.517*** | 0.113*** | 0.244*** |
(0.04) | (0.08) | (0.04) | (0.08) | |
Ln(R&D) | 0.010* | 0.028*** | 0.026*** | 0.025*** |
(0.01) | (0.01) | (0.01) | (0.01) | |
Ln(researchers) | 0.032 | 0.028*** | 0.089* | 0.155* |
(0.04) | (0.01) | (0.05) | (0.08) | |
scale | -0.50*** | 0.050 | -0.220*** | -0.361*** |
(0.14) | (0.19) | (0.07) | (0.11) | |
University FE | Yes | Yes | Yes | Yes |
Year FE | Yes | Yes | Yes | Yes |
IV | Yes | Yes | Yes | Yes |
Adjusted | 0.899 | 0.813 | 0.894 | 0.801 |
Observations | 4550 | 4550 | 4155 | 4155 |
Note: The dependent variable is the number of inventions and utilities. The estimation is conducted using a negative binomial model. ***p < 0.01, **p < 0.05, *p < 0.1.
The Role of Social Networks
Another key determinant of patent transfers is the social network strength of university researchers, particularly their collaborations with firms and industry partners. Universities that maintain strong connections with enterprises and other external stakeholders are more likely to engage in efficient knowledge transfer and technology commercialization. Stronger networks facilitate trust-building, reduce information asymmetry, and enhance the matching process between technology suppliers (universities) and demand-side firms.
To measure social network strength, this study uses the proportion of university R&D funding sourced from enterprises and other external organizations. Universities with above-median industry funding shares are classified as having strong social networks, while those below the median are categorized as having weak social networks.
The regression results in Table 8 confirm that strong social networks amplify the effect of patent liquidity on innovation, particularly for invention patents. In universities with strong social networks, a one-standard-deviation increase in patent liquidity leads to a 23.1% increase in invention patent output and a 29.2% increase in utility model output. By contrast, in universities with weaker social networks, the corresponding effects on invention and utility model patents are 10.1% and 32.3%, respectively.
Table 8. The Heterogeneous Effects of Patent Liquidity on Innovation by Social Networks | ||||
Strong Social Networks | Weak Social Networks | |||
Inventions | Utilities | Inventions | Utilities | |
Liquidity | 0.231** | 0.292*** | 0.101*** | 0.323*** |
(0.04) | (0.09) | (0.04) | (0.08) | |
Ln(R&D) | 0.014*** | 0.013** | 0.024*** | 0.042*** |
(0.00) | (0.01) | (0.01) | (0.01) | |
Ln(researchers) | 0.084* | 0.108 | 0.031 | 0.126* |
(0.05) | (0.08) | (0.05) | (0.07) | |
scale | -0.176 | -0.708 | -0.061 | -0.316*** |
(0.15) | (0.20) | (0.07) | (0.11) | |
University FE | Yes | Yes | Yes | Yes |
Year FE | Yes | Yes | Yes | Yes |
IV | Yes | Yes | Yes | Yes |
Adjusted | 0.893 | 0.790 | 0.758 | 0.723 |
Observations | 5195 | 5195 | 3510 | 3510 |
Note: The dependent variable is the number of inventions and utilities. The estimation is conducted using a negative binomial model. ***p < 0.01, **p < 0.05, *p < 0.1.
These findings suggest that universities with stronger industry ties are more effective at leveraging patent market activity for innovation. Institutions with well-established corporate collaborations may receive more market feedback, align their research more closely with industry needs, and benefit from faster and more efficient technology diffusion. The weaker effect of social networks on utility model patents suggests that these patents, which often require less industry-specific customization, are less reliant on strong external networks for commercialization. The results underscore the importance of both researcher qualifications and social network strength in shaping the relationship between patent liquidity and university innovation. Universities with highly qualified researchers and strong external links are better positioned to capitalize on an active patent market, making targeted policies crucial for enhancing university technology transfer.
Policymakers should consider strategies such as providing commercialization training for researchers, establishing more structured university-industry collaboration programs, and developing market-oriented research incentives to further bridge the gap between academic innovation and market application. Strengthening institutional support for technology transfer offices (TTOs) and reducing barriers to industry-academia partnerships could also enhance the innovation impact of university patent liquidity.
Conclusion
This study investigates the landscape of university patent transfers, including the extent of university participation, the composition of transferred patent types, and their industry distribution. Building on this, it further explores the impact of patent transfers on university innovation. The findings reveal a steady increase in the number of university patent transfers over time, with the probability of post-grant transfers also showing an upward trend. The majority of transferred patents belong to the invention category, with a significant portion associated with the manufacturing sector. More importantly, patent transfers exert a positive effect on university innovation, with a one-standard-deviation increase in patent liquidity leading to a 12.5% rise in innovation output.
The effects of patent transfers on innovation, however, are not uniform across different types of patents and universities. Universities with higher-qualified researchers and stronger social networks experience a greater innovation boost from patent liquidity, while institutions located in regions with higher economic development and better financing accessibility also benefit more significantly from technology transfer activities. These findings contribute to the existing literature on university technology transfer and patent transactions, providing theoretical and empirical support for policies aimed at optimizing university patent commercialization. The results suggest that patent transfers not only activate existing technological resources and generate economic value but also stimulate further innovation, reinforcing the dynamic role of universities in driving technological progress.
To enhance the efficiency and impact of university patent transfers, universities should strengthen their technology transfer infrastructure, aligning with the policy framework established by the Ministry of Science and Technology and the Ministry of Education to advance professional technology transfer institutions. This involves establishing dedicated technology transfer offices (TTOs), cultivating specialized personnel, and improving commercialization mechanisms. Additionally, universities should develop effective incentive structures to encourage researchers to actively participate in technology transfer, incorporating commercialization performance as a key metric in faculty evaluation and promotion decisions. Given the high degree of information asymmetry and market uncertainty in patent transactions, universities must also implement robust risk assessment frameworks to mitigate potential challenges in the commercialization process. Furthermore, leveraging patent navigation services can provide universities with strategic insights into industry trends, facilitating the development of high-value patents and improving the alignment of research outputs with market needs.
By strengthening the institutional mechanisms that support technology transfer, universities can further enhance their role in innovation ecosystems, improve the efficiency of knowledge commercialization, and contribute more effectively to technological advancement and economic development.
References
- Bozeman, B., & Gaughan, M. (2007). Impacts of grants and contracts on academic researchers’ interactions with industry. Research Policy, 36(5), 694–707.
- Buenstorf, G., & Geissler, M. (2012). Not invented here: Technology licensing, knowledge transfer, and innovation based on public research. Journal of Evolutionary Economics, 22(3), 481–511.
- Buenstorf, G., & Schacht, A. (2013). We need to talk; or do we? Geographic distance and the commercialization of technologies from public research. Research Policy, 42(2), 465–480.
- Chapple, W., Lockett, A., & Siegel, D. (2005). Assessing the relative performance of U.K. university technology transfer offices: Parametric and non-parametric evidence. Research Policy, 34, 369–384.
- Fang, V. W., Tian, X., & Tice, S. (2014). Does stock liquidity enhance or impede firm innovation? The Journal of Finance, 69(5), 2085–2125.
- Figueroa, N., & Serrano, C. J. (2019). Patent trading flows of small and large firms. Research Policy, 48(7), 1601–1616.
- Galasso, A., Schankerman, M., & Serrano, C. J. (2013). Trading and enforcing patent rights. The RAND Journal of Economics, 44(2), 275–312.
- Grandi, A., & Grimaldi, R. (2005). Academics’ organizational characteristics and the generation of successful business ideas. Journal of Business Venturing, 20(6), 821–845.
- Han, P., Liu, C., & Tian, X. (2022). Does trading spur specialization? Evidence from patenting. SSRN Working Paper.
- Hochberg, Y. V., Serrano, C. J., & Ziedonis, R. H. (2018). Patent collateral, investor commitment, and the market for venture lending. Journal of Financial Economics, 130(1), 74–94.
- Hsu, P. H., Tian, X., & Xu, Y. (2014). Financial development and innovation: Cross-country evidence. Journal of Financial Economics, 112(1), 116–135.
- Jensen, R., & Thursby, M. (2003). The academic effects of patentable research. NBER Working Paper.
- Lach, S., & Schankerman, M. (2008). Incentives and inventions in universities. RAND Journal of Economics, 39(2), 403–433.
- Markman, G. D., Gianiodis, P. T., & Phan, P. H. (2004). Entrepreneurship from the ivory tower: Do incentive systems matter? The Journal of Technology Transfer, 29(3), 353–364.
- Markman, G. D., Gianiodis, P. T., & Phan, P. H. (2005). Innovation speed: Transferring university technology to market. Research Policy, 34(7), 1058–1075.
- Powers, J. B., & McDougall, P. (2005). Policy orientation effects on performance with licensing to start-ups and small companies. Research Policy, 34(7), 1028–1042.
- Serrano, C. J. (2010). The dynamics of the transfer and renewal of patents. RAND Journal of Economics, 41(4), 686–708.
- Serrano, C. J. (2018). Estimating the gains from trade in the market for patent rights. International Economic Review, 59(4), 1877–1904.
- Serrano, C. J., & Ziedonis, R. (2019). How redeployable are patent assets? Evidence from failed startups. Academy of Management Proceedings, 2019(1).
- Shane, S. (2001). Technological opportunities and new firm creation. Management Science, 47(2), 205–220.
- Siegel, D. S., Waldman, D., & Link, A. (2003). Assessing the impact of organizational practices on the relative productivity of university technology transfer offices: An exploratory study. Research Policy, 32(1), 27–48.
- Siegel, D. S., Waldman, D. A., & Atwater, L. E. (2003). Commercial knowledge transfers from universities to firms: Improving the effectiveness of university-industry collaboration. Journal of High Technology Management Research, 14(1), 111–133.
- Siegel, D. S., Waldman, D. A., & Atwater, L. E. (2004). Toward a model of the effective transfer of scientific knowledge from academicians to practitioners: Qualitative evidence from the commercialization of university technologies. Journal of Engineering & Technology Management, 21(1–2), 115–142.
- Thursby, M., Thursby, J., & Gupta-Mukherjee, S. (2007). Are there real effects of licensing on academic research? A life cycle view. Journal of Economic Behavior and Organization, 63(4), 577–598.
- Tian, X., & Xu, J. (2022). Do place-based policies promote local innovation and entrepreneurship? Review of Finance, 26(3), 595–635.
- Weckowska, D. M. (2015). Learning in university technology transfer offices: Transactions-focused and relations-focused approaches to commercialization of academic research. Technovation, 41/42, 62–74.
- Xu, Z., Parry, M. E., & Song, M. (2011). The impact of technology transfer office characteristics on university invention disclosure. IEEE Transactions on Engineering Management, 58(2), 212–227.
Zhenyu Gong
Central University of Finance and Economics, School of Innovation and Development, China Academy of Management and Economics, Beijing, China, 100060.